Earlier this month we hosted a DYNA (Dyalog North America) event. We returned to the same venue as DYNA24, and again had a capacity crowd.
The day started with an update from our CTO, Morten Kromberg, regarding the latest developments at Dyalog Ltd – both within the company and with Dyalog and its associated offerings. One notable change is a transition in how we will provide the documentation. From Dyalog v20.0, we are moving towards using open-source markdown-based documentation on GitHub. The benefits of this approach are improved searching functionality and the ability for more people, internal and external, to contribute. If you see an issue with the documentation, you can raise a GitHub issue or even submit a correction as a pull request.
Several new tools are in progress, including:
- an interface to Apache Kafka, which is a popular open-source distributed event streaming platform used by thousands of companies.
- a tool to perform static analysis of APL code that will detect vulnerabilities and other bad practices. This will help our customers adhere to “best practices” for software vulnerability analysis.
- leveraging AI both to help our customers develop APL code and to help them use AI in their own applications.
Morten then spoke about the current and forthcoming versions of Dyalog, sharing the highlights of v19.0, reviewing v19.4.1(!), and previewing v20.0, which will be available later this year. Among its many features are two highly anticipated ones:
- Array notation – this will have a significant impact on how arrays can be specified in code. Creating namespaces is now particularly easy, as rather than creating a namespace and subsequently assigning values in the namespace, they can be completely specified in notation.
- Inline tracing – this will allow the developer to trace each element of an APL expression, displaying information about left and right arguments, operands, and so on. This should help make understanding long or dense lines of APL code much easier.
Morten’s second presentation focused on efforts to provide sets of migration tools for two different purposes – to migrate applications that are currently using Dyalog’s ⎕WC
to non-Windows platforms, and to migrate applications written in other APL implementations to Dyalog APL.
EWC (Everywhere Window Create) is intended to be as syntactically identical to ⎕WC
as possible to simplify the process of enabling existing ⎕WC
applications to run on platforms other than Microsoft Windows with virtually no changes. EWC uses HTML, JavaScript, and CSS in the HTMLRenderer or a browser to render the GUI. This opens up the possibility for users to integrate other JavaScript libraries, inject custom CSS, or develop custom controls to add additional GUI elements beyond those that ⎕WC
can provide alone. Neil Kirsopp is our resident JavaScript expert, and is leading the client side of EWC. Morten showed screenshots of an existing ⎕WC
application and its EWC equivalent, as well as a new EWC application that he developed to help track the administrative work necessary for maintenance and scheduling of the sailing ship he co-owns.
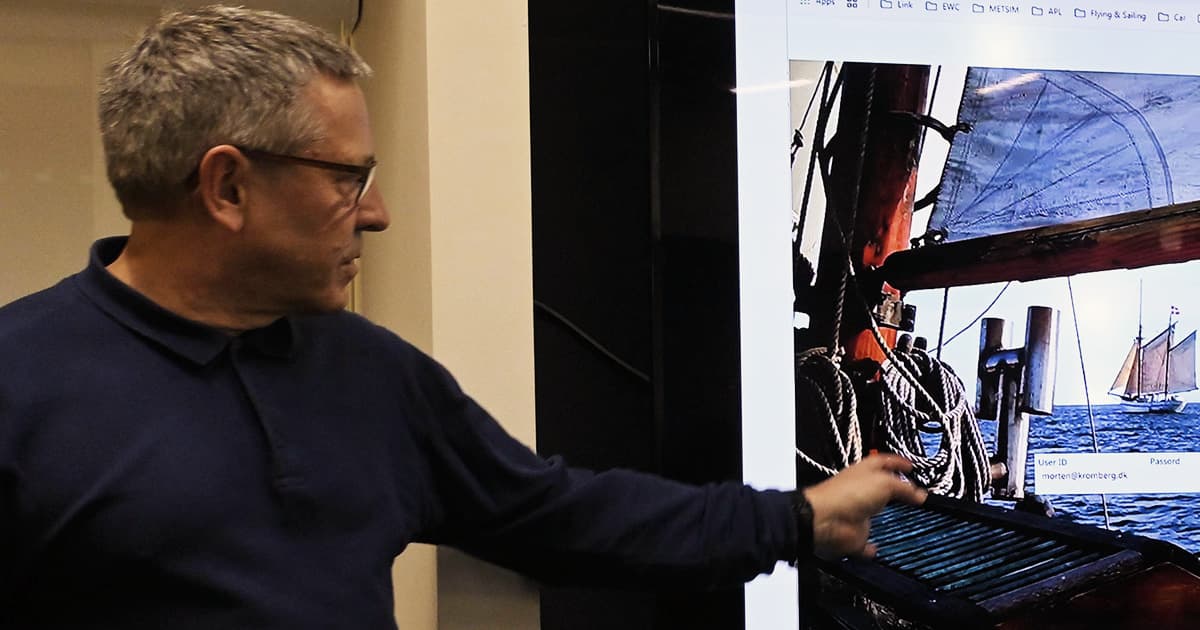
Photo by Devon McCormick
Morten next discussed source code migration from APL+Win to Dyalog APL. The challenge of migrating a “living” application where changes are being made to the APL+Win code while it is also being converted to Dyalog APL has resulted in a methodology using code in parallel text files, using Git for source code management. A framework to translate the syntactic incongruities between the two APLs has been developed. Perhaps the most daunting difference lies in the GUI architectures. APL+Win uses ⎕WI
where Dyalog APL uses ⎕WC
. A new tool that we have developed to ameliorate this is ∆WI
, which is a ⎕WI
emulator that uses ⎕WC
under the covers. Although work is not yet complete, the pilot customer is very pleased with the migration effort and the performance of ∆WI
.
Morten also noted that many ∆WI
-based applications can run using EWC and, therefore, should be able to run in the cloud. Davin Church (Creative Software Design), with his extensive APL+Win experience as well as his Dyalog experience, has been enlisted to help develop ∆WI
. At this point, Davin joined Morten and mentioned that when he compared Dyalog’s APL-based equivalents to APL+Win’s C-based “fastfns”, the Dyalog code typically ran slightly faster.
Mark Wolfson (BIG) spoke about how Dyalog is used within his company. BIG has revolutionised the Jewelry industry, and their product is known for accommodating and handling many different input files and formats from jewellery shops across the USA. Mark is quick to advocate for, and experiment with, some of the newest Dyalog features. Now with Jarvis and EWC, he has been able to very quickly deploy new front-end features to his customers, sometimes sometimes even while they are describing their problem to him over the phone! This is in stark contrast to trying to create a ticket to get the same features built and created by the C# development team.
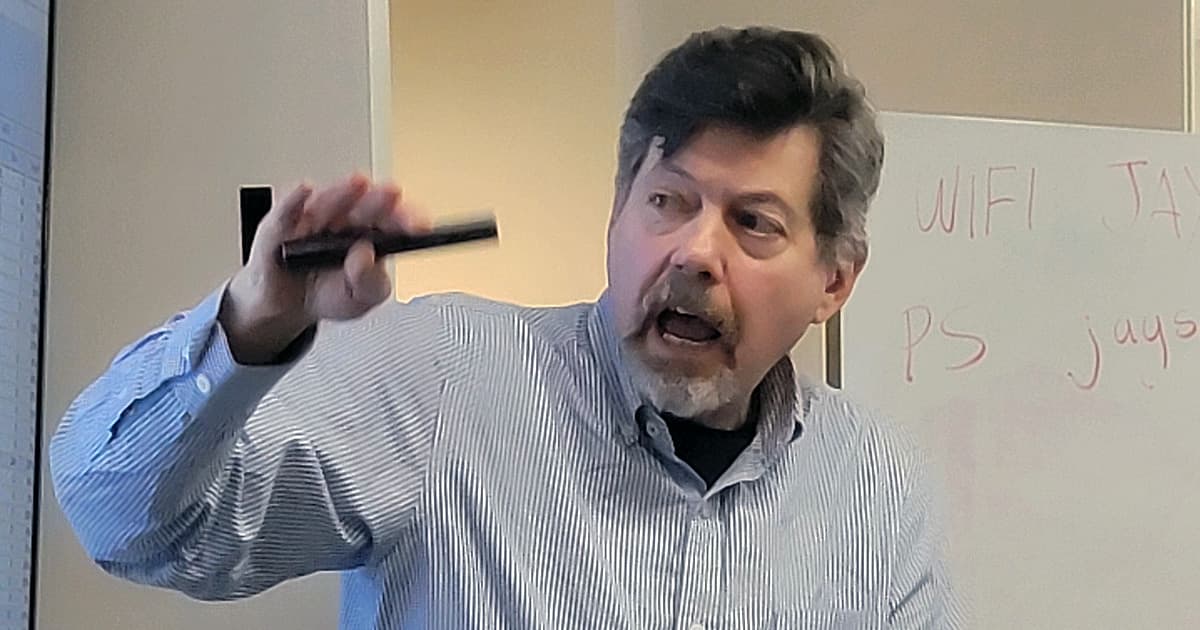
Brian Becker then presented “The Many Faces of Jarvis”. Jarvis is Dyalog’s web service framework that can be used to deliver JSON or REST-based web services – it provides a mechanism for clients to access your APL code over the net without them having to know anything about APL. Since its inception, Jarvis has become a key component of many Dyalog projects, both internally and amongst our customers. Brian described how features have been added to Jarvis in direct response to customer needs.
Josh Davis showcased some of the tools and functionality that are used in day-to-day development. He decided to live dangerously, and demonstrated this by working out a problem in real time using some of the public datasets made available by New York City. He did an analysis and produced a report of tree data (the plant, not the datatype!) across the five boroughs. It involved the use of some newer primitives such as the key and at operators, other system functions for reading/writing files, the HTMLRenderer and a JavaScript plotting library, COM for Microsoft Windows automation, .NET for cryptography routines, SQAPL for database access, HttpCommand for web requests, and ⎕R
for regular expressions support. All these tools come with a Dyalog installation.
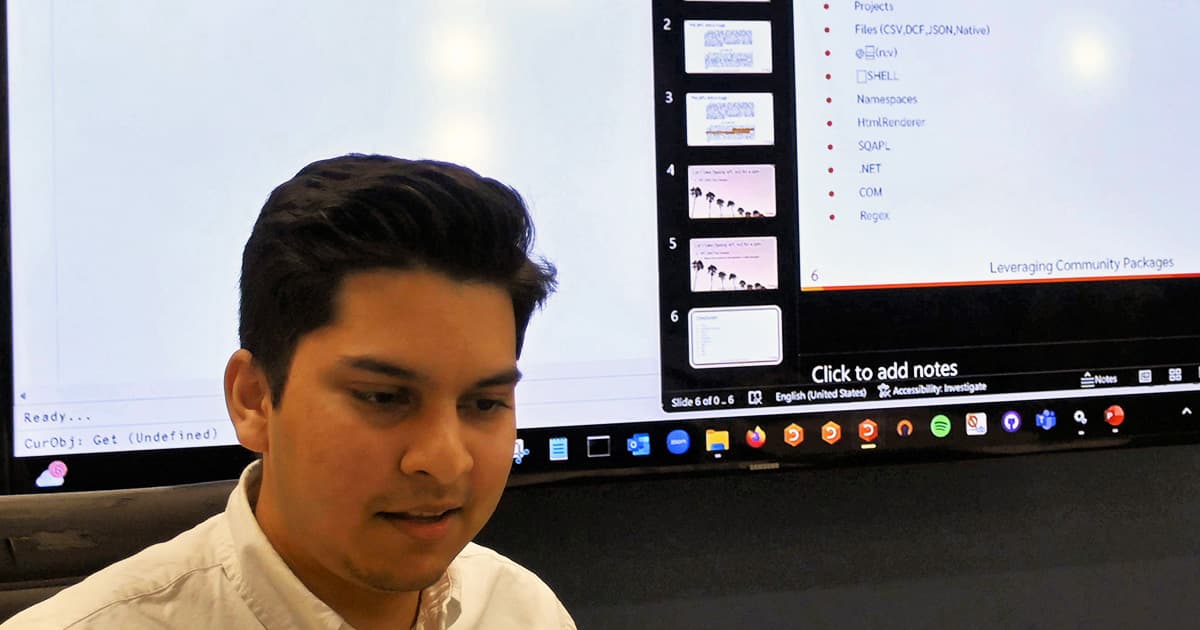
Photo by Devon McCormick
Max Sun (BCA Research) presented a demonstration of some of the tools he used to build the talk he gave at LambdaConf last year; he runs APL examples in a Jupyter Notebook, using SharpPlot for plots.
Brian and Mark presented thoughts and developments on Dyalog and AI. Brian posed two questions:
- How can Dyalog use AI to help our customers?
- Making documentation easier to search and navigate
- Explaining what a piece of APL code is doing
- Generating APL code
- What can Dyalog do to help our customers use AI?
- What tools can we provide to help our customers to integrate AI into their applications
Integrating APL and AI faces several challenges. Firstly, there’s very little APL with which to train Large Language Models (LLMs), especially compared to languages like Python and JavaScript. This can lead to frequent “hallucinations”, where the LLM just makes something up. Secondly, APL is not a focus for AI purveyors. Although there is documentation and packages for Python and other language to interface to LLMs, it’s up to us to develop them for APL.
Brian demonstrated a Google NotebookLM, into which much of the Dyalog documentation had been uploaded. This provided “context” for the LLM, which made the search results more accurate and applicable. Next came a demonstration of Cursor, which is essentially Anthropic’s Claude LLM integrated within Visual Studio Code. Brian provided a dfn and asked what the code did. The response was impressively accurate. He then loaded the HttpCommand git repository and asked for a summary – again the results were impressive. Brian then showed how he asked an LLM to write a simple piece of APL code. The LLM got it wrong, which Brian pointed out. This went through several iterations, with the LLM profusely apologising for its mistakes as it went on to generate another, different, mistake! It should be noted that different LLMs will perform better or worse depending on what body of material they have been trained on, and new, better, LLMs are being rolled out all the time.
Brian has used HttpCommand to develop interfaces to OpenAI‘s and Anthropic’s APIs. Many LLMs are OpenAI compatible, which means their API is very similar to OpenAI’s. Developing and documenting interfaces to LLMs will continue to be a focus. Mark discussed how AI could be useful at BIG, citing real-world use cases to balance inventory, improve look-ups, and provide alternative items based on their attributes and photographic similarity. Mark also raised the question of using commercial cloud-based AI for proprietary data. How private and secure is your intellectual property?
Brian’s presentation on “The APL Ecosystem” highlighted the online resources that exist to help people learn APL and participate in, and contribute to, the APL community. The single most useful resource seems to be the APL Wiki, but there is also TryAPL, APLCart, APL videos on YouTube, APL-related GitHub repositories, Hacker News, the APL Orchard on Stack Exchange, the APL Farm on Discord, the APL Challenge, the APL Forge, and various APL-related presentations at LambdaConf, Functional Conf, and PLDI.
To close the event, Diane Hymas asked everyone to “remember how you felt the day you discovered APL”. This was her lead-in to introducing The APL Trust – a non-profit entity aiming to promote awareness and use of the APL programming language by funding APL projects, especially in the areas of STEM (Science, Technology, Engineering, and Mathematics). Although the initial plan was to have one global fund, for tax purposes it was more expedient to start with a US fund, with other related funds in the UK and EU to follow. Hopefully the APL Trust will become an incubator of some great new APL ideas and software!
After the day’s scheduled agenda, most of the attendees walked around the corner to the Yard House for dinner and drinks. All in all, the day was fun, informative, and a great opportunity to interact with other APL enthusiasts.
————————————–
All the presentation materials from DYNA Spring 2025 can be downloaded.
DYNA Fall 2025 will take place on 29-30 September – register for updates as information becomes available.